Introduction
The artificial intelligence landscape is undergoing rapid transformation as AI becomes increasingly embedded across business operations. This integration is not only streamlining workflows and enhancing productivity but also driving substantial investor interest and a surge in M&A deal activity. AI companies that deliver tangible efficiency gains are reshaping competitive dynamics and commanding premium valuations, often surpassing those of traditional technology firms.
However, valuing AI businesses presents unique challenges, distinct from the well-trodden paths of SaaS and other software models. As a result, understanding AI valuation requires a new analytical framework. In this article, we explore the intricacies of valuing AI companies, compare them with conventional models, and share our perspectives on AI valuation.
For a deeper dive into current AI valuation multiples, please refer to our detailed analysis here: AI Valuation Multiples 2025
What’s Driving AI Acquisitions?
The surge in AI-related acquisitions is fueled by a range of strategic motivations, as companies across industries race to harness the transformative power of artificial intelligence. Rather than relying solely on internal development, acquiring AI firms offers a faster, more effective path to staying competitive. Key drivers include:
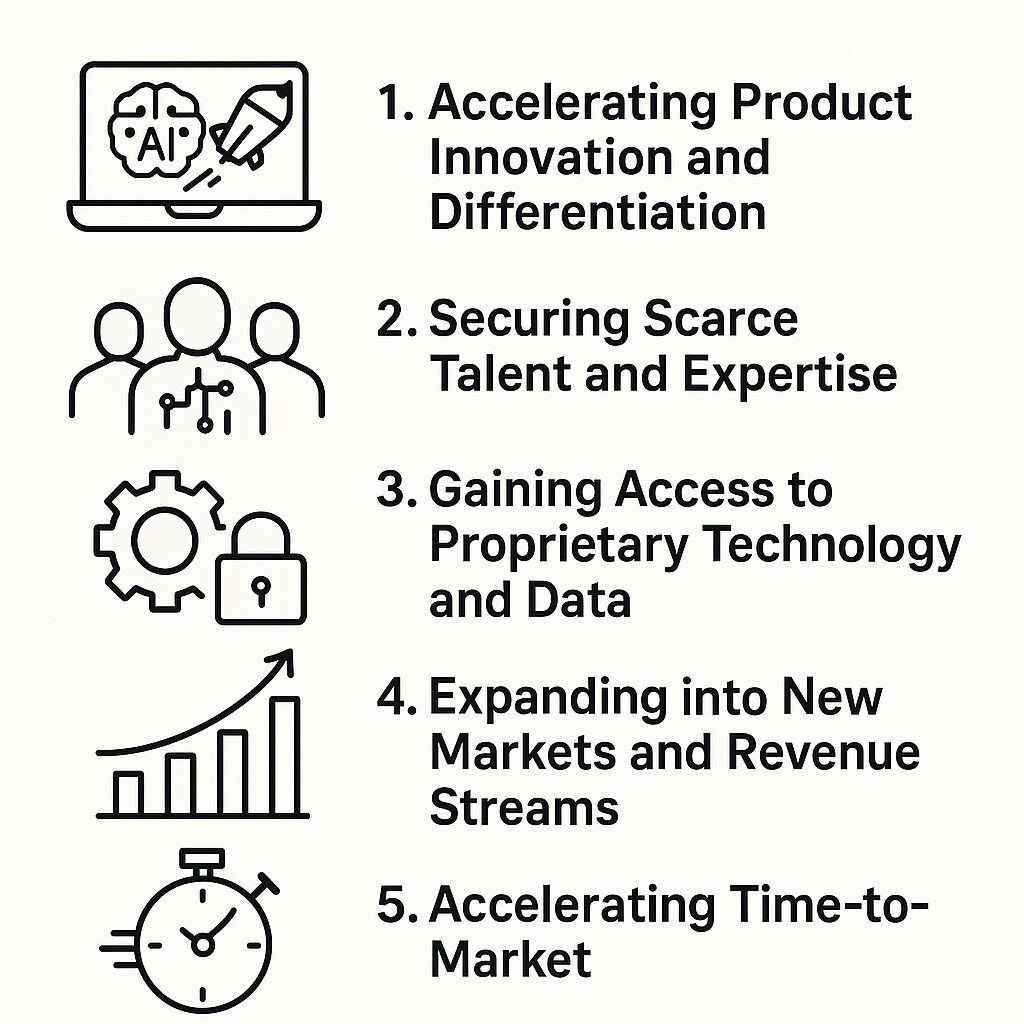
1. Accelerating Product Innovation and Differentiation
- Acquiring AI capabilities allows companies to embed intelligent features into existing products and services—enhancing performance, enabling personalization, and unlocking entirely new use cases. This not only improves customer experience but also strengthens market positioning in increasingly digital and data-driven environments
2. Securing Scarce Talent and Expertise
- The AI talent pool remains limited, with intense global competition for top engineers, data scientists, and researchers. Acquisitions offer a fast track to onboarding experienced teams, often with a proven track record of innovation and execution
3. Gaining Access to Proprietary Technology and Data
- Many AI firms have developed unique algorithms, models, or curated datasets that underpin their competitive advantage. Acquiring these assets can provide a technological edge that would be difficult—and time-consuming—to replicate organically
4. Expanding into New Markets and Revenue Streams
- AI can serve as a catalyst for growth, enabling businesses to enter adjacent markets or launch entirely new product lines. Strategic acquisitions help accelerate this expansion by bringing in domain-specific AI capabilities that align with the acquirer’s broader growth strategy
5. Accelerating Time-to-Market
- In the rapidly evolving AI landscape, speed matters. M&A often provides a more agile route to innovation than in-house R&D, helping companies keep pace with emerging trends and shifting consumer expectations
How AI Companies Differ from Traditional SaaS Companies
While AI companies may share some surface-level similarities with traditional SaaS businesses, their underlying economics, competitive dynamics, and valuation drivers are fundamentally distinct. These differences require a more tailored approach to valuation, reflecting the nuanced realities of the AI business model.
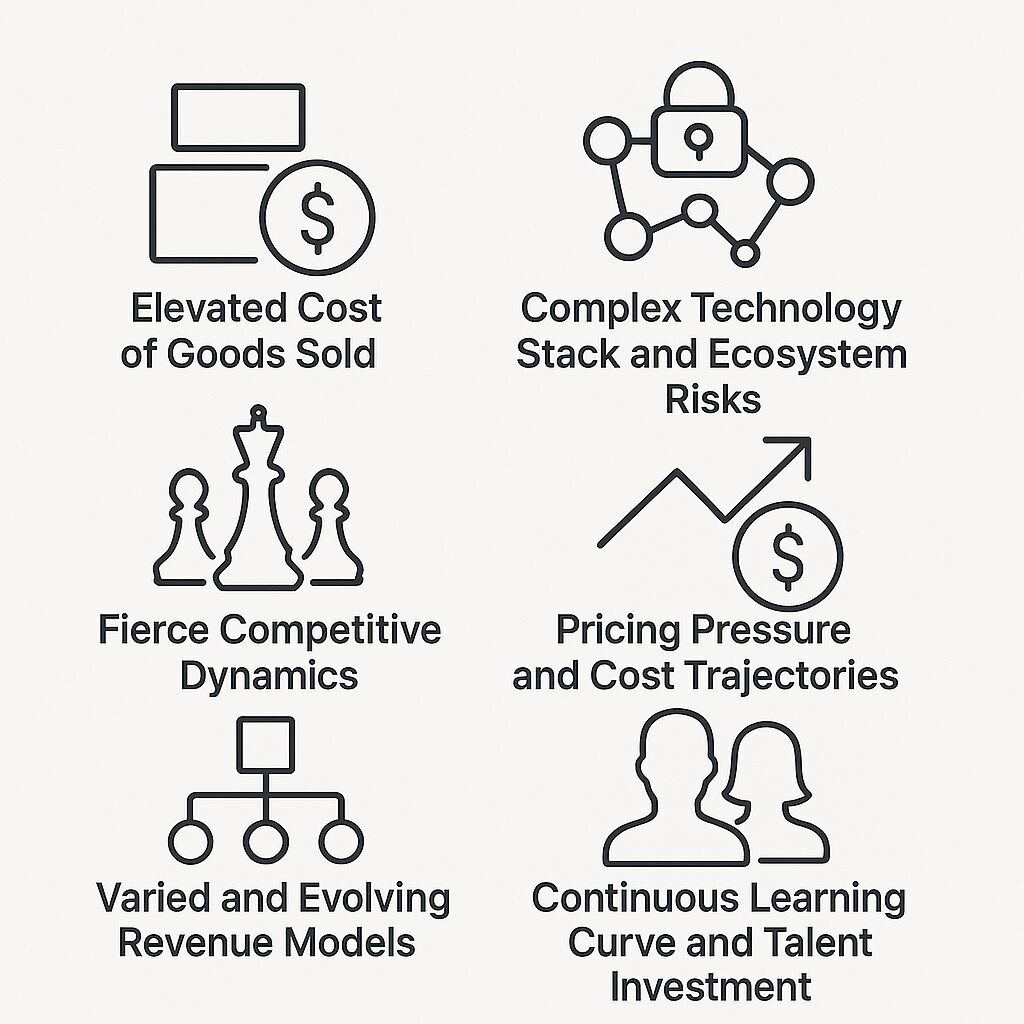
1. Elevated Cost of Goods Sold (COGS)
- Traditional SaaS companies benefit from low marginal costs once software is deployed. In contrast, AI businesses, particularly those built on large language models (LLMs) or generative AI, often incur significant variable costs. Each query processed by external models like OpenAI’s GPT or Anthropic’s Claude may come with measurable inference costs, driving up COGS and compressing margins in ways not typically seen in SaaS
2. Complex Technology Stack and Ecosystem Risks
- AI systems are built on layered, interconnected frameworks involving data pipelines, model training, cloud infrastructure, and API integration. This complexity introduces both scalability opportunities and operational risks, including data privacy, security vulnerabilities, and potential model instability, that can impact both valuation
3. Fierce Competitive Dynamics
- The pace of innovation in AI is relentless, with companies racing to develop proprietary models or build differentiated applications atop foundational models. This arms race leads to heightened R&D spend, reduced visibility on long-term winners, and pressure on pricing power, all of which complicate projections of future cash flow and sustainable market leadership
4. Pricing Pressure and Cost Trajectories
- While AI inference costs may decline over time due to hardware improvements and model optimization, this trend may also drive down prices for AI services and API access. Valuation expectations must incorporate assumptions about margin compression and the potential commoditization of certain AI capabilities over time
5. Varied and Evolving Revenue Models
- Unlike the predictable subscription revenue common in SaaS, AI companies often monetize through a blend of usage-based APIs, licensing agreements, tiered consumption pricing, and even model-as-a-service offerings. This diversity requires a granular understanding of usage patterns, customer retention, and cost-to-serve in order to accurately model recurring revenue potential
6. Continuous Learning Curve and Talent Investment
- AI businesses must continuously invest in cutting-edge research and workforce education to remain competitive. This includes not only engineering talent, but also domain experts, data scientists, and infrastructure specialists. The high pace of innovation demands a culture of rapid iteration and adaptation, further differentiating AI firms from more stable SaaS operations
Key Valuation Challenges
Valuing AI companies presents a distinctive set of challenges that go beyond traditional software or SaaS frameworks. The sector’s breakneck pace of innovation, evolving business models, and cost variability require a more dynamic, forward-looking valuation methodology. Below are the key complexities acquirers must contend with:
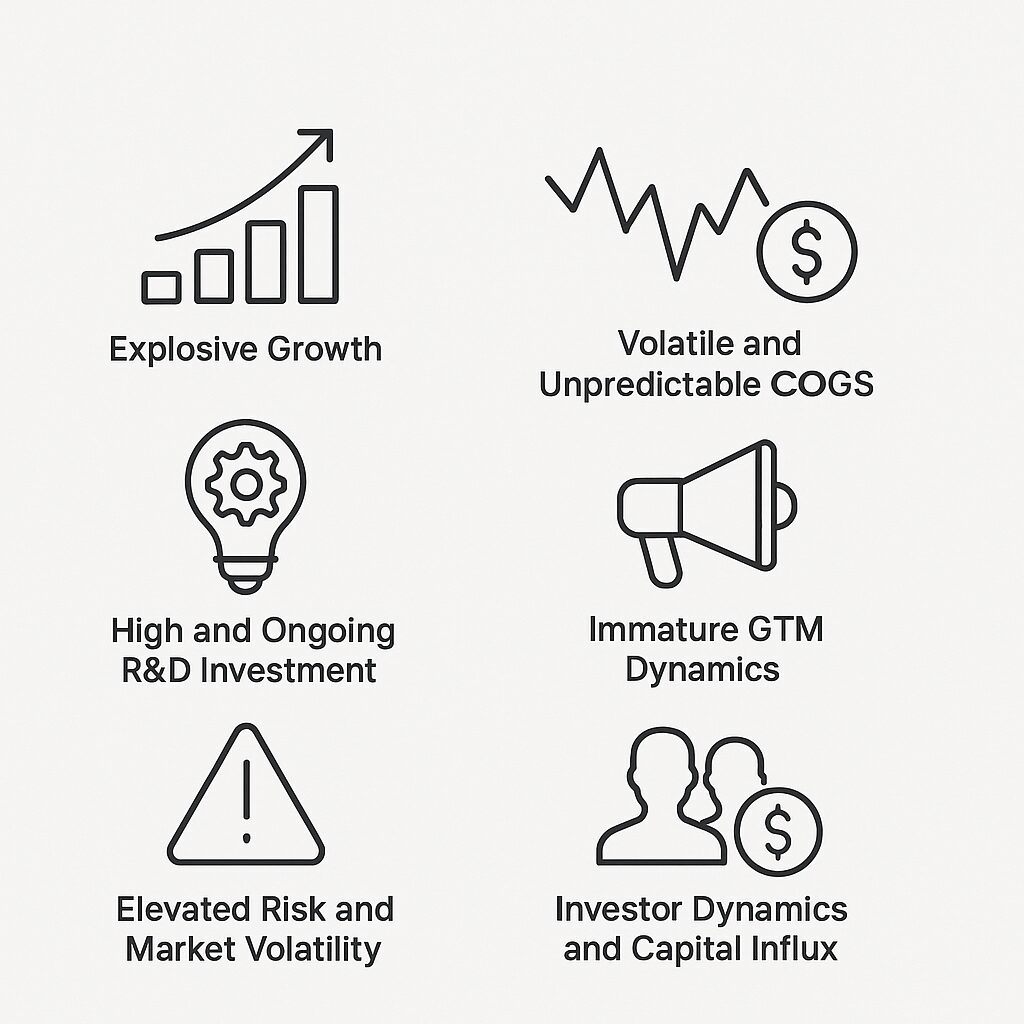
1. Explosive Growth
- Many AI companies exhibit exponential revenue growth, often fueled by novel use cases and early market enthusiasm. However, these businesses frequently lack established operating histories or proven paths to profitability. Their models are still maturing, making it difficult to assess long-term sustainability and cash flow visibility
2. Volatile and Unpredictable COGS
- Unlike SaaS firms with stable delivery costs, AI companies—particularly those dependent on external large language models (LLMs)—face variable inference costs tied to usage volume and provider pricing. These costs are influenced by rapid shifts in model architecture, efficiency gains, and licensing terms, complicating accurate gross margin forecasting
3. High and Ongoing R&D Investment
- Staying at the forefront of AI requires substantial, ongoing R&D spending. Hiring elite AI researchers and engineers, training proprietary models, and refining infrastructure demands significant capital allocation. These costs are structural rather than optional, often consuming a large share of revenue in early-stage companies
4. Immature Go-to-Market (GTM) Dynamics
- Conventional SaaS metrics such as Customer Acquisition Cost (CAC) and Lifetime Value (LTV) are harder to apply in AI contexts. GTM strategies are still experimental in many cases, with evolving pricing models, unclear customer usage patterns, and limited historical churn data. This uncertainty complicates the modeling of customer economics and scalability
5. Elevated Risk and Market Volatility
- The combination of intense competition, rapid technological obsolescence, and regulatory uncertainty elevates the risk profile of AI companies. Investors must contend with the possibility that today’s cutting-edge application could be commoditized or leapfrogged tomorrow
6. Investor Dynamics and Capital Influx
- The AI sector has seen a flood of strategic and financial investors—from venture capital to big tech—chasing breakout opportunities. While this capital injection has fueled innovation and growth, it has also inflated valuations, especially in early-stage companies with limited revenue history. Understanding how competitive investor dynamics impact valuation benchmarks is essential
Our View on Valuing AI Companies
Valuing AI companies is inherently complex. With limited historical M&A precedent and rapidly evolving business models, comparables are often unreliable. Yet despite the hype and uncertainty, one principle remains constant: the intrinsic value of any company is the present value of its expected future cash flows.
For that reason, a discounted cash flow (DCF) approach should anchor any robust valuation, even in the face of limited visibility. While SaaS multiples can provide a reference point—particularly for AI firms with recurring revenue—they must be applied cautiously. Key differences in COGS structure (due to inference costs) and elevated R&D intensity significantly alter financial profiles.
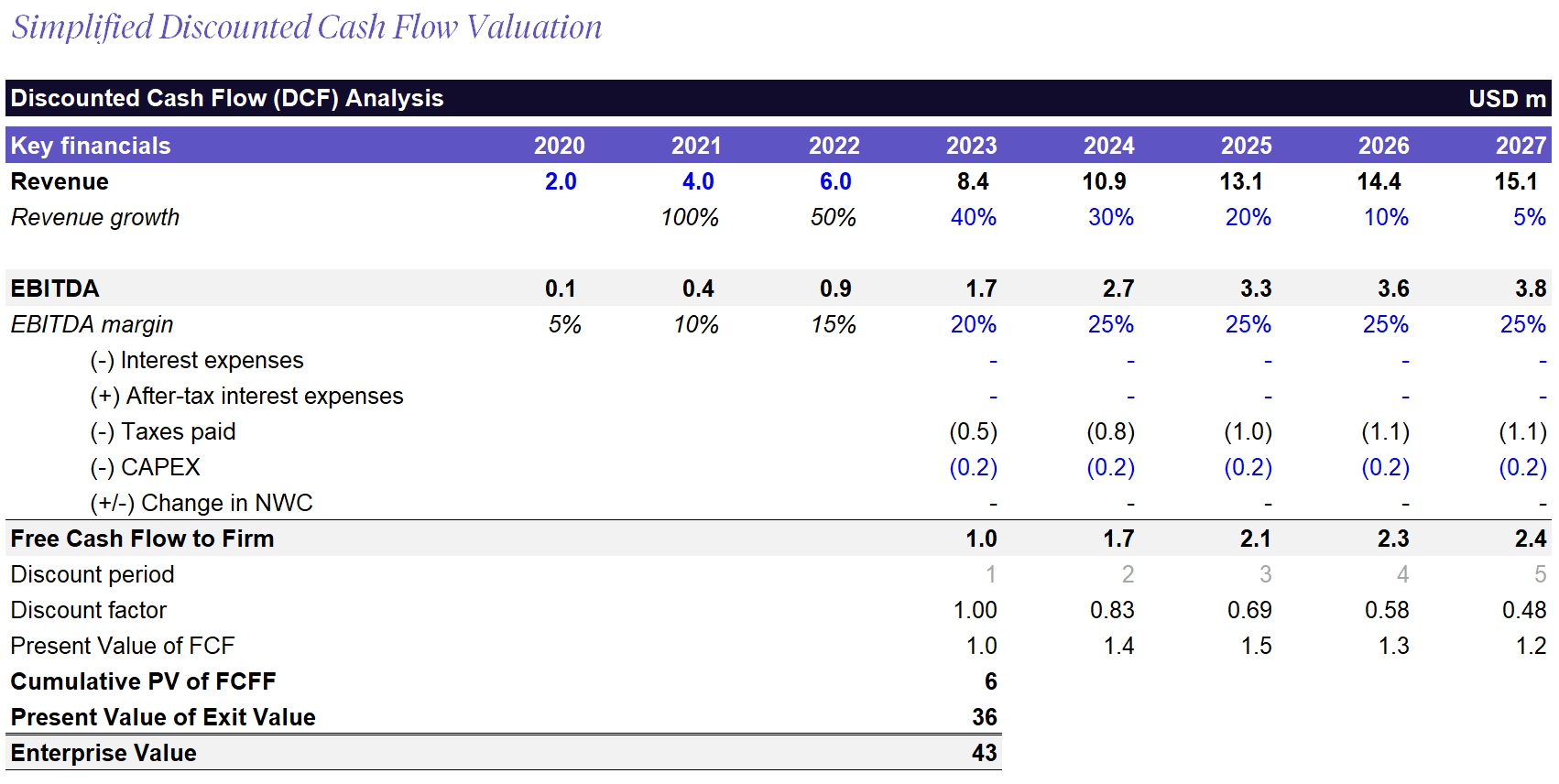
As AI companies mature and scale, focus should shift toward sustainable economics: defensible margins, monetization strategy, infrastructure efficiency, and response to evolving risks, such as security and model drift. High-profile valuations (e.g., OpenAI, Perplexity) are often outliers, shaped by strategic dynamics that don’t reflect the broader market.
Ultimately, each AI business must be assessed on its unique fundamentals—including product application, technical differentiation, market opportunity, and team quality. AI is redefining how businesses operate, and valuation frameworks must evolve accordingly.
Why you should Partner with a Technology M&A Advisor when Selling your AI company
Every AI company is unique, just as every founder’s journey is unique. Therefore, it’s crucial to seek guidance from experts in the AI M&A space, particularly M&A advisors who specialize in the technology sector and can understand the fundamentals of your specific situation.
Technology M&A advisors possess deep knowledge of industry dynamics, valuation methodologies, and the intricacies of the M&A process. While you focus on managing your business, advisors diligently ensure that every detail is addressed and advocate for the best possible deal on your behalf. Technology M&A advisors success is intertwined with yours, and their expertise and management of the sale process can often significantly influence the final sale price.
About Aventis Advisors
Aventis Advisors is an M&A advisor focusing on technology and growth companies. We believe the world would be better off with fewer (but better quality) M&A deals done at the right moment for the company and its owners. Our goal is to provide honest, insight-driven advice, clearly laying out all the options for our clients – including the one to keep the status quo.
Get in touch with us to discuss how much your business could be worth and how to maximize the valuation.